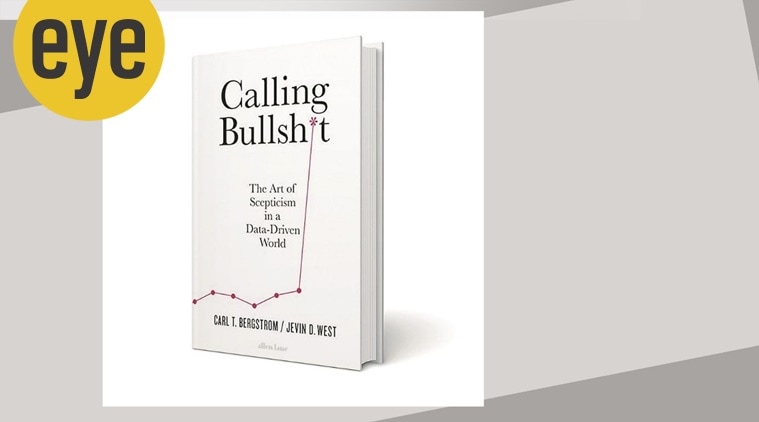
This engaging book, which breaks the news that the numbers do not speak for themselves as advertised, but as they are tweaked, is due out next Tuesday. But the publishers should have rushed it to press months ago when the pandemic broke out and graphs based on dubious data, depicting the progress of the disease, the utter disarray of the public health response, the chances that you would be infected, and the slim subset of the chance that you would die, began to appear every day on the front pages. Contexts kept shifting, the conclusions reliably different and united only in their ability to urge you to lose faith in data. West and Bergstrom, who teach information science at the University of Washington, remind us that faith is old hat. To know what exactly is going on, you must be able to evaluate the data, and its manipulation, for yourself. It’s surprisingly easy.
When we were in high school, statistics and probability were sniffed at as inexact mathematical fields that rely on the p-value, a standard that is often manipulated. Unless you wished to study economics and see the world, you didn’t waste time on them. Mean, median, mode, standard deviation, permutations and combinations, a ritual nod to Pascal, and you moved on. To Boolean algebra, if computers fascinated you, and to trigonometry and calculus for everything else. Who could have thought that statistics would turn out to be the most important skill for understanding what is going on in human affairs?
In the age of Big Data and Machine Learning, the problem appears to be amplified by the sheer size of datasets and the inscrutability of algorithms. A movement seeks transparency in algorithms — if you have been passed over by a computer, you should know why — but the aim is easier stated than achieved. Machine learning is trained on datasets which are classified by humans, and it writes a program to categorise future data. But even the authors of a system may not know exactly how that works. The book refers to an ML system tasked with separating pictures of huskies and wolves. But it was looking at the background, not the animals. The AI had realised that while huskies may be shot in various human contexts, wild wolves are most likely to be photographed against a background of snow. It was only looking at the background, and spuriously but accurately identifying the animals.
Besides, most algos are proprietary, for a good reason. If Google released its ranking algorithm publicly, it would spark off a global arms race as everyone and their teenage nephew tried to game it. But the authors remind us that generally, it is not necessary to climb into the black box in which the algo lives. Analysing the quality of input and output only takes plain logic, and serves the purpose.
Let us return to the coronavirus and the squiggles and diagrams on the front page and in explainers mapping its devastating journey through the human race. For wild inconsistency, consider the bizarre debacle concerning the need for masks, with opinion veering, like the sweep of a windshield wiper, between their utter uselessness and their critical role in containment. Most embarrassingly, the WHO, which has always set the global agenda sensibly, fuelled the uncertainty, undermining public trust in the authorised version, and in the reliability of science itself.
And then there was this farrago (pace Tharoor) of graphs, charts and data visualisations. Here, too, the authors offer simple checks. Does the scale begin from zero, the point of origin of perspective, or an arbitrary number which conveniently tweaks apparent results? Is the scale linear, or does 1 cm represent one year at first and 10 years afterwards, steepening curves? Is the timescale zoomed out to the extent that critical changes become invisible? The authors object strenuously to sexing up graphical representations, for instance by illustrating a farm to fork story by using the tines of a fork, out of scale, to represent data. Humans are highly visual animals, and tweaking a graph is the easiest way to lead them astray.
The book makes a distinction between old-school BS, which only conveys the impression that something is seriously being done about something which seriously bothers you (in 1980s India, “immediate implementation of an action plan on a war footing, under the direct oversight of high-powered committee headed by retired Supreme Court judge”) and new-school BS, which uses “the language of math and science and statistics to create the impression of rigour and accuracy.” It is so pervasive that calling it out responsibly must become a public duty.
Recipients of propaganda believe the first only if they are politically inclined to, but are helpless in the face of the latter. The species believes itself to be numerically challenged, and abjectly surrenders when it is confronted by data, no matter how obviously spurious or misleading it may be. Sadly, the numbers never spoke for themselves. Now, twisted data has become so pervasive that fact-checkers, the indomitable Gauls of the information age, cannot stem the tide on their own any more. It is time we all went digital plogging.