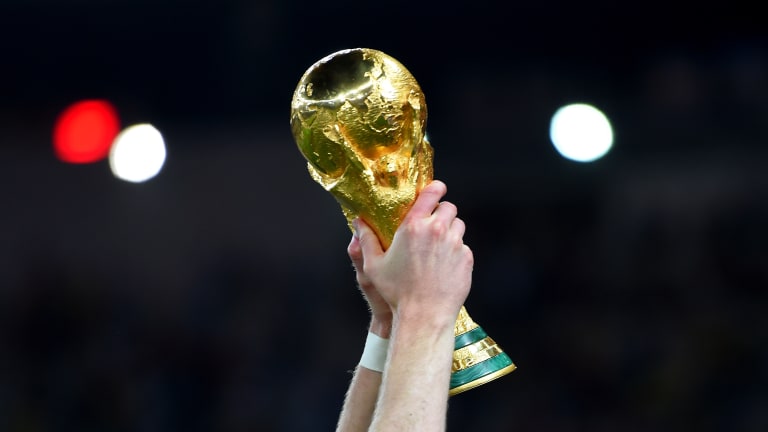
When Lionel Messi observed that “in football...talent and elegance mean nothing without rigour and precision”, he was clearly thinking as much about the econometrics behind forecasting the score as the tactics behind winning the match. But while selecting the best starting eleven requires human judgment and experience, choosing the best variables to predict the outcome of a game is better left to a statistical model. Or more precisely, 200,000 models: harnessing recent developments in “machine learning,” data was mined on team characteristics and individual players to work out which factors help to predict match scores. This gave a large number of forecasts, which were combined to produce an overall projection. This was then simulated 1,000,000 possible evolutions of the tournament to gauge the probability of each team progressing through the rounds.
The key predictions are:
*Brazil will win its sixth World Cup title—defeating Germany in the final on July 15th
*France has a higher probability than Germany of winning the World Cup. But its (bad) luck in the draw sees it meeting Brazil at the semi-final stage, and the team may not be strong enough to make it past Seleção.
*Those looking for a repudiation of Gary Lineker’s observation that “football is a simple game; 22 men chase a ball for 90 minutes and, at the end, the Germans win” will be disappointed: Germany is forecast to defeat England in the quarter finals on July 7.
*Spain and Argentina are expected to underperform, losing to France and Portugal in the quarter finals, respectively.
*Despite the traditional boost that comes with hosting the competition, Russia just fails to make it through the group stage .
Football and Machine Learning: From Nottingham Forest to Random Forest
We are drawn to machine learning models because they can sift through a large number of possible explanatory variables to produce more accurate forecasts than conventional alternatives.
More specifically, we feed data on team characteristics, individual players and recent team performance into four different types of machine learning models to analyse the number of goals scored in each match. The models then learn the relationship between these characteristics and goals scored, using the scores of competitive World Cup and European Cup matches since 2005. By cycling through alternative combinations of variables, we get a sense of which characteristics matter for success and which stay on the bench. We then use the model to predict the number of goals scored in each possible encounter of the tournament and use the unrounded score to determine the winner. For example, Germany narrowly beats England in the quarterfinals with 1.47 vs 1.28 goals.
We group together several team-level and player-level variables for ease of exposition. Four characteristics stand out. Team-level results are the most important driver of success. Recent team performance — measured with the “Elo” rankings — accounts for about 40 per cent of overall explanatory power.
But even after taking team performance into account, individual players make a difference. We find that player-level characteristics — including the average player rating on the team, as well as attacking and defending abilities — add another 25 per cent of explanatory power. Recent momentum — as measured by the ratio of wins to losses over the past ten matches — matters.
Similarly, the number of goals scored in recent games and the number of goals conceded by the opponent team help gauge success in the next game.
Why Brazil are favs
Brazil is clearly the strongest team across these metrics, with the highest Elo rating, talented individual players and a good win/lose ratio in recent games. We also see why France and Germany run neck and neck for second: Germany has a higher Elo rating than France, but France has performed better in recent games. And France appears to have a more unfavorable draw than Germany: if France and Germany started in each other’s respective group, the most likely result would be a Brazil-France final (although the winner would remain Brazil).
Spain’s chances are likewise diminished by a tough draw, facing Portugal in the group, and needing to get past France and Brazil in the knock-out stages. Finally, Argentina ranks higher on Elo than Portugal, but loses to Portugal in the quarter finals due to poor performance in recent games.
How confident can we be?
It is difficult to assess how much faith one should have in these predictions. We capture the stochastic nature of the tournament carefully using state-of-the-art statistical methods and we consider a lot of information in doing so (including player-level data). But the forecasts remain highly uncertain, even with the fanciest statistical techniques, simply because football is quite an unpredictable game. This is, of course, precisely why the World Cup will be so exciting to watch.
Source: Goldman Sachs Global Investment Research